TL;DRThe results of our second Protein Design Competition show dramatic progress in the field. Out of 400 tested proteins, 378 expressed successfully (95%) and 53 of these bound to their target EGFR (14% success rate). This represents a 5x improvement in binding success compared to just three months ago. The best designs even matched or exceeded the performance of Merck's Cetuximab, a clinically approved therapeutic antibody.
The competition demonstrated that protein design is becoming more reliable and accessible - 30 out of 130 participants managed to create at least one successful binder using various approaches, from optimizing existing antibodies to designing completely new proteins from scratch.
While we can now generate functional proteins more consistently, a key challenge remains: predicting which designs will work best before testing them in the lab.
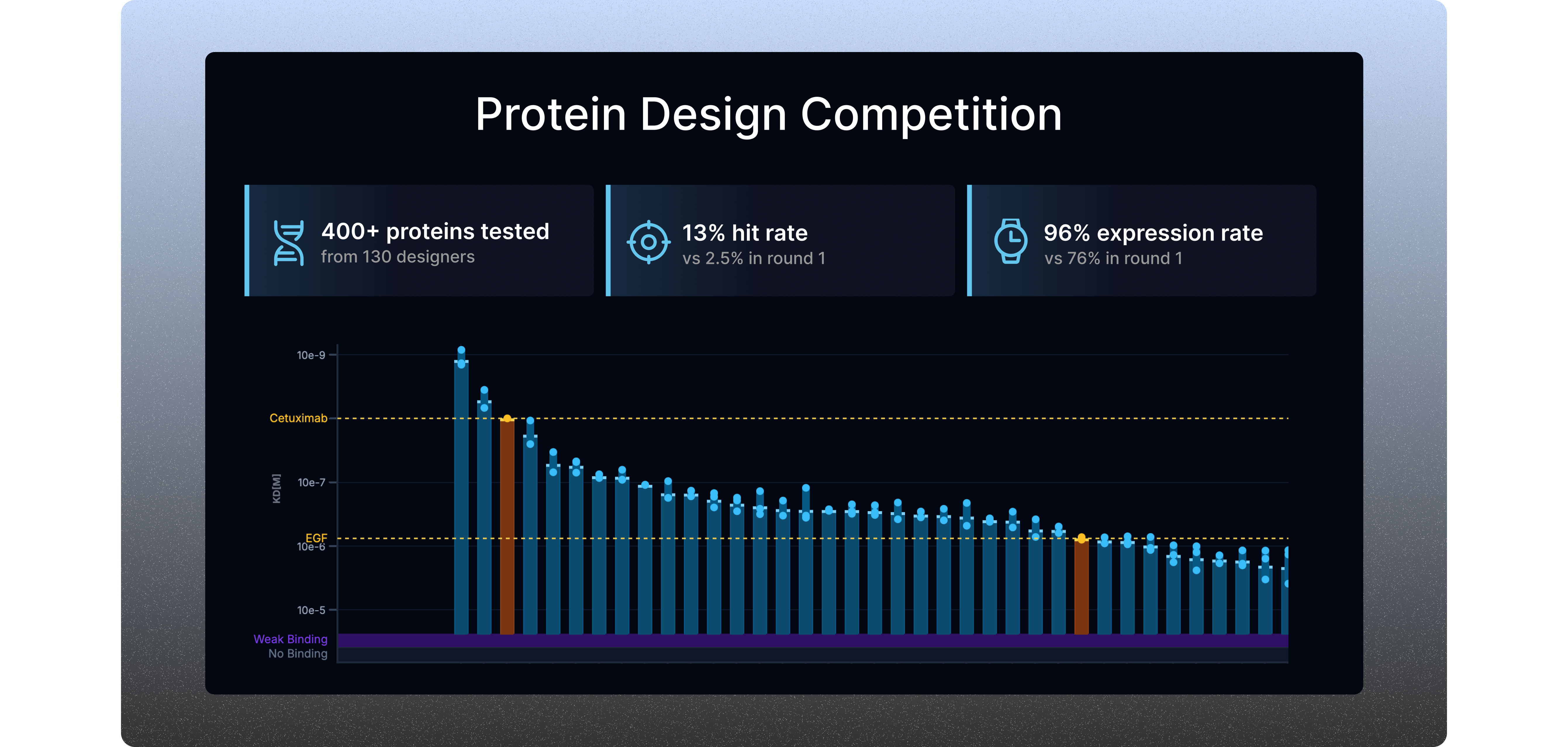
We released the results of our second Protein Design Competition just about 2 weeks ago… and they were great!
A quick recap of what this was all about:
- We tasked designers to create new protein binders for EGFR, an important binding target for therapeutic proteins.
- We then ranked them based on a weighted score of AlphaFold2’s ipTM and iPAE metrics (scoring how well AlphaFold “likes” the interface) and ESM2 pseudolikelihood (ESM2 PLL), a measure of how “natural” a sequence looks like given ESM2 learned distribution.
- From these, we chose 400 sequences to be tested experimentally in our lab: the top 100 as determined by the rankings and then another 300 handpicked by us at Adaptyv, where we included at least one protein from each designer and then tried to maximize the diversity of interesting models and design strategies.
And the designers did not disappoint. In our previous analysis here, we went through how the meta shifted from the first round, in particular how close the race was in the last few days as some submitters tried to increase their chances of landing in the top 100 spots.
We had also given you some guidance regarding which hotspots to target on EGFR, which models to use to improve your binder’s expression chances, and which models to use if you’re truly interested in venturing into the de novo world. We believe these guidelines, and the community momentum that was bubbling up the scenes, with “protein design recipes”, blog posts, and general advice, were the causal factors that led to an increase in both hit rates and expression: from 2.5% for the former to 13%, and from 76% to 96%. These figures are something to be proud of, and we would like to thank all participants for joining, getting involved in the community, and especially those who were actively writing about their methods. We believe these nuggets of info will be valuable to move the field of binder design forward.
After all this preamble, let’s outline what this blog post will cover: We’ll first recognize the winners: the top 3 designers as ranked by binding affinity and a special prize for the de novo designs. The top 3 designers had the chance to present their workflows at the recent NeurIPS AIDrugX workshop. Then, we will go through a rapid-fire round of data analysis.
Spoiler: we’ll publish a paper that will go more in-depth into both rounds and what we’ve learned from them. If you want to join in on this effort (either by writing about the method you used, making some nice figures, or just debating with participants about statistical analysis and confounding factors), and if you took part in one of the rounds, please fill out this form here. Thus we’re keeping our analysis short and snappy here, focusing on only the second round, but there is more to investigate!
Lastly, you can download all binding data from the first and second rounds. Tag us and let us know if you have done any analysis on this and have some cool findings!
Meeting the winners
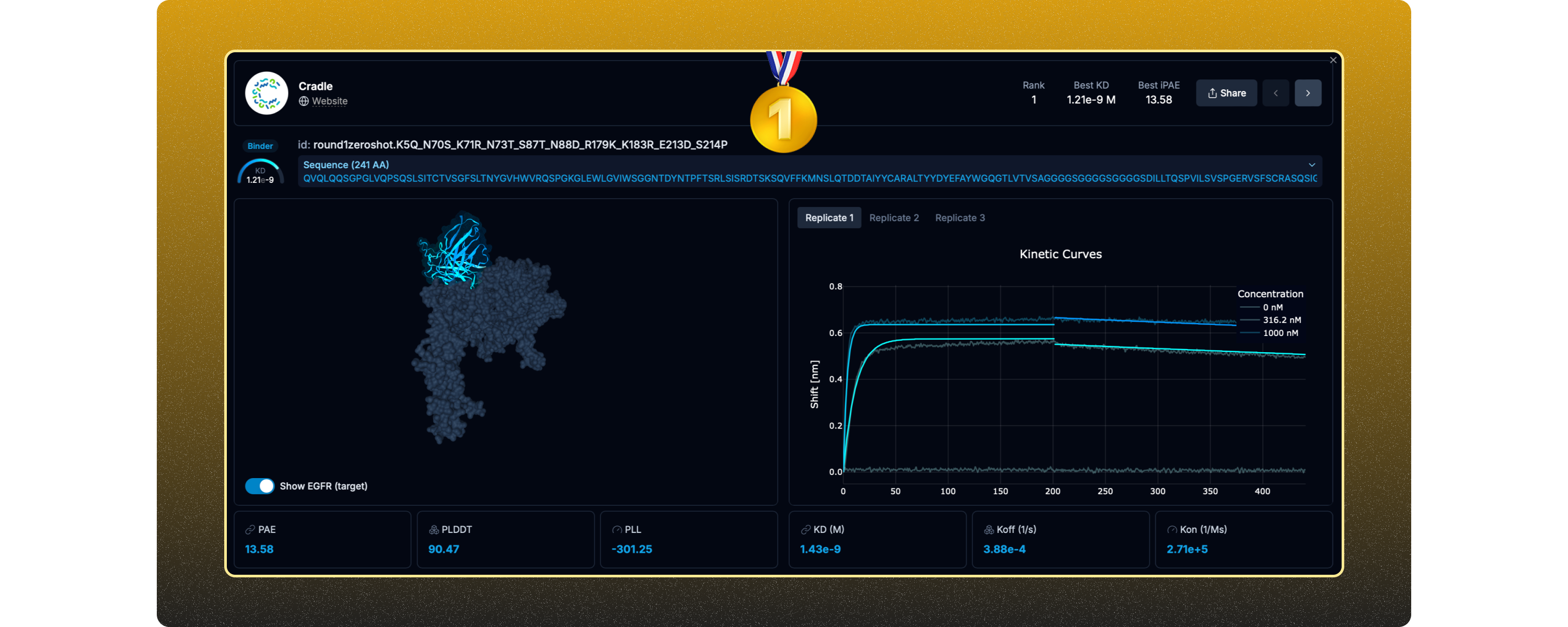
The number 1 spot was taken by Cradle and their optimized Cetuximab variant.
Designed in ”about 30 minutes and over some kombucha”, it highlights the strength of Cradle’s platform to optimize existing proteins for a set of biologically relevant properties, binding affinity in this case. As mentioned in their design process notes, they used a zero-shot approach without training their model on any labelled data. This yielded an improvement of Merck’s Cetuximab, by making exactly 10 stabilizing mutations in the framework regions (”FRs” as opposed to “CDRs”, the complementarity-determining regions, which are highly variable and form the typical antibody binding loops that determine their specificity).
Similar approaches to induce loop-stabilizing mutations in framework regions have been proven effective before. Moreover, recent advances in antibody engineering propose modulating the Fc regions as well, resulting in improved half-lives, as opposed to the current approaches of Fab changes (including FRs and CDRs) to modulate affinity and specificity. So there is a wider potential in changing or improving an antibody’s function than only CDR engineering, and Cradle demonstrated it in this competition to great success.
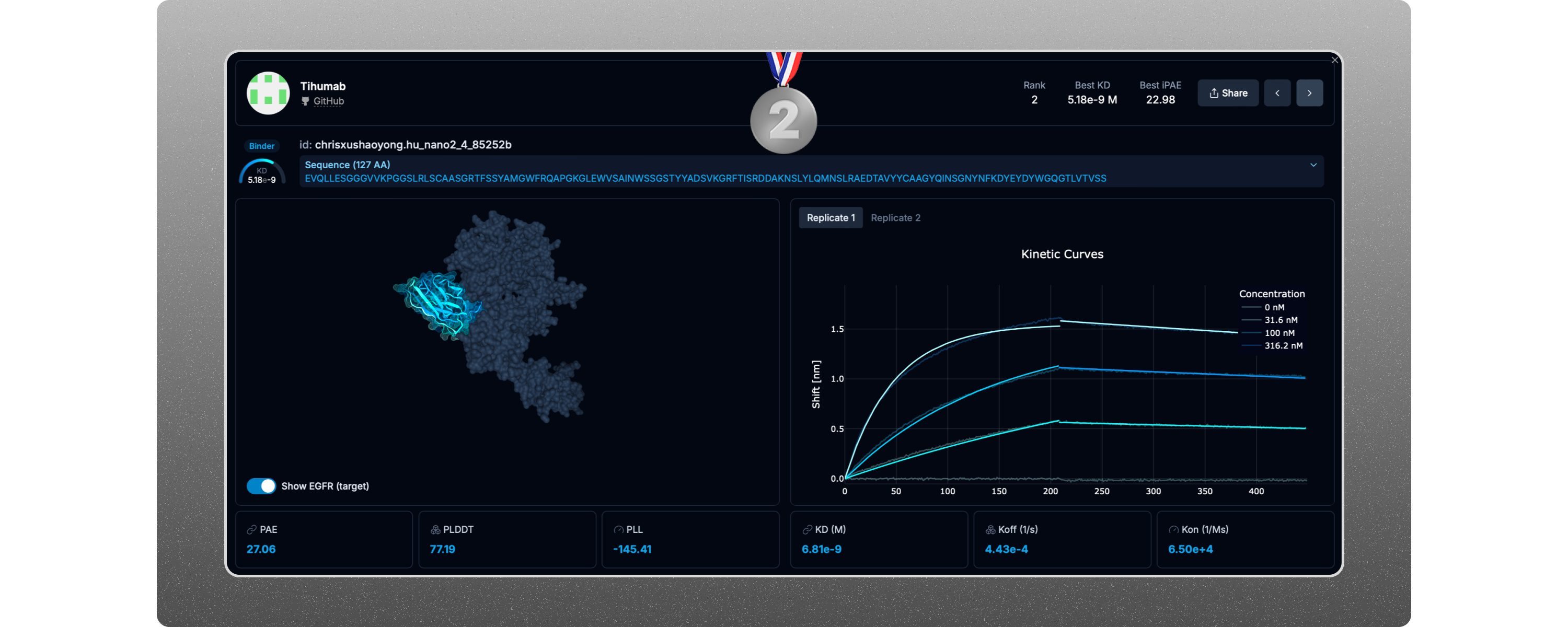
A optimized nanobody takes the number 2 spot. Designer Chris Xu mentions that his workflow involves CDR grafting, in which the FR regions are replaced with homologues human germline ones, followed by optimization of CDR stability and hydrophobicity, followed by developability filtering, with metrics for “humanness” and immunogenicity. Despite not ranking high in the leaderboard as his approach did not directly optimize for them, we are very excited that a lot of people approached our challenge from a similar perspective: not only designing a binder, but aiming to also optimize other therapeutic properties at the same time.
In terms of binding affinity, Chris’ nanobody is also on par with Cetuximab. Even more interestingly, his approach yielded two other binders, one with a binding affinity in the tens of nM range, and one in the hundreds. We’d love to see more experiments on those designs to see if their optimized developability properties hold up in the lab!
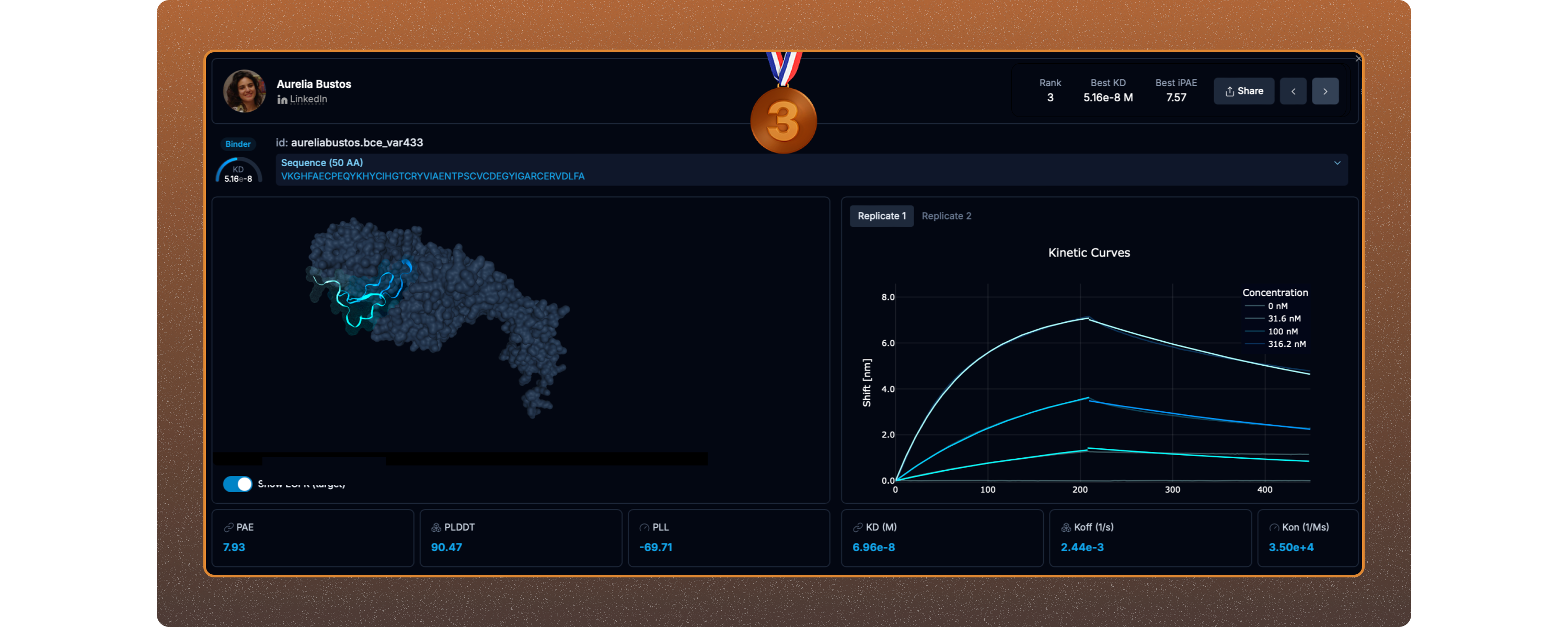
Our 3rd spot was claimed by Aurelia Bustos and her TGF$\alpha$ optimization approach. We’ve highlighted this method before, and you can find a more detailed explanation from her as well. Briefly, she started from TGF$\alpha$, masked out the binding region, then used it to scaffold the non-binding backbone using RFdiffusion. She followed up with ProteinMPNN inverse folding and trimming the excess residues to maximize ESM2’s log-likelihood. Thus, her resulting sequence is 50 amino acids in length, likely due to the unnormalized likelihood score we have been using.
This achieved the best of both worlds in this competition: several of her designs ranked in the top 20 as they optimized the metrics and a lot were good binders. 8 of her submissions bound, in fact, which results in an 80% success rate, with some in the tens, hundreds of nM, or mM ranges. Almost all had better binding affinities than the EGF control. However, we did not have a TGF$\alpha$ control to compare and see if the affinities also improved compared to the natural ligand.
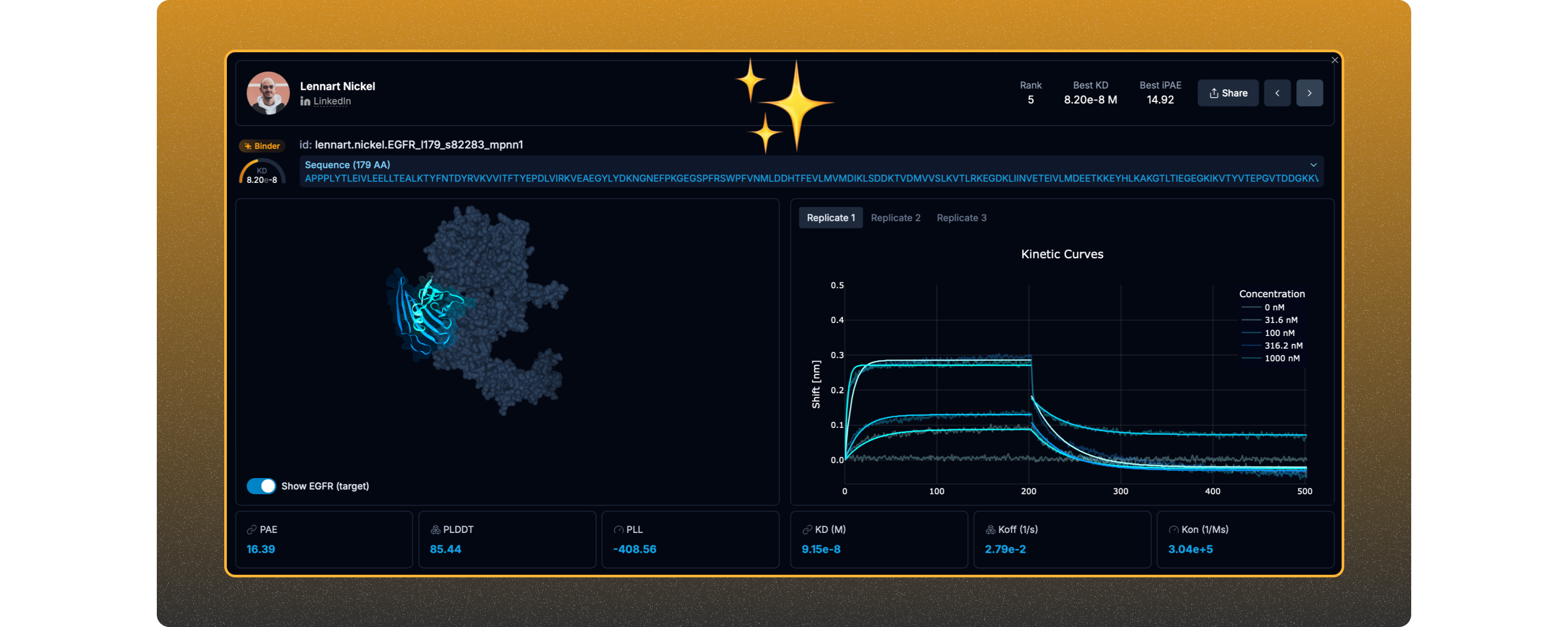
The best de novo designed protein comes from Lennart Nickel & Martin Pacesa using BindCraft.
If you paid attention, you will have noticed that the top 3 spots were dominated by redesigned binders, from known antibody therapeutics, to nanobodies and binders from nature. Yet, a lot of participants wanted to push their strategies to their limits by generating something completely brand new, a de novo binder. Here
For this, BindCraft seemed to be the favorite approach: The model generates a de novo binder by sampling a noisy initial state, and increase the chances for landing in the top 100 by optimizing (via gradient descent) for metrics like iPAE and ipTM. We noticed that it wasn’t as successful at landing in the top 100 spot compared to directed evolution, trimming excess amino acids, or other methods. We speculate it was due to other metrics like ESM2’s likelihood that were not directly accounted for in the model and that it was optimizing for multiple other properties (like compactness and number of interface contacts).
Lennart Nickel & Martin Pacesa wanted to make their design task even harder by asking BindCraft to create $\beta$-sheet binder, introducing a helicity-penalizing loss. You can see that they achieved this and a good binding affinity of 91.5 nM. There is yet another impressive achievement for BindCraft in this round: it claimed 6 out of the 7 leaderboard spots won by de novo binders, with the remainder being designed with RFdiffusion and ProteinMPNN.
At least for this competition, BindCraft and hallucination seem to have dethroned diffusion models due to their increased hit rates, one of the greatest meta change we’ve observed from Round 1 just a few months ago to today. We also think there is a huge avenue for customizing BindCraft and making the design task more challenging (e.g., losses with fewer interface contacts, more tunable physicochemical properties of the interface, targeting more difficult, hydrophilic sites, scaffolding, or including pre-trained models to tune both specificity and affinity, or making the overall process less computationally demanding). We’re incredibly excited to see how this nifty design tool will evolve in the future!
Now, onto some data analysis!
How many binders and expressed designs did we see?
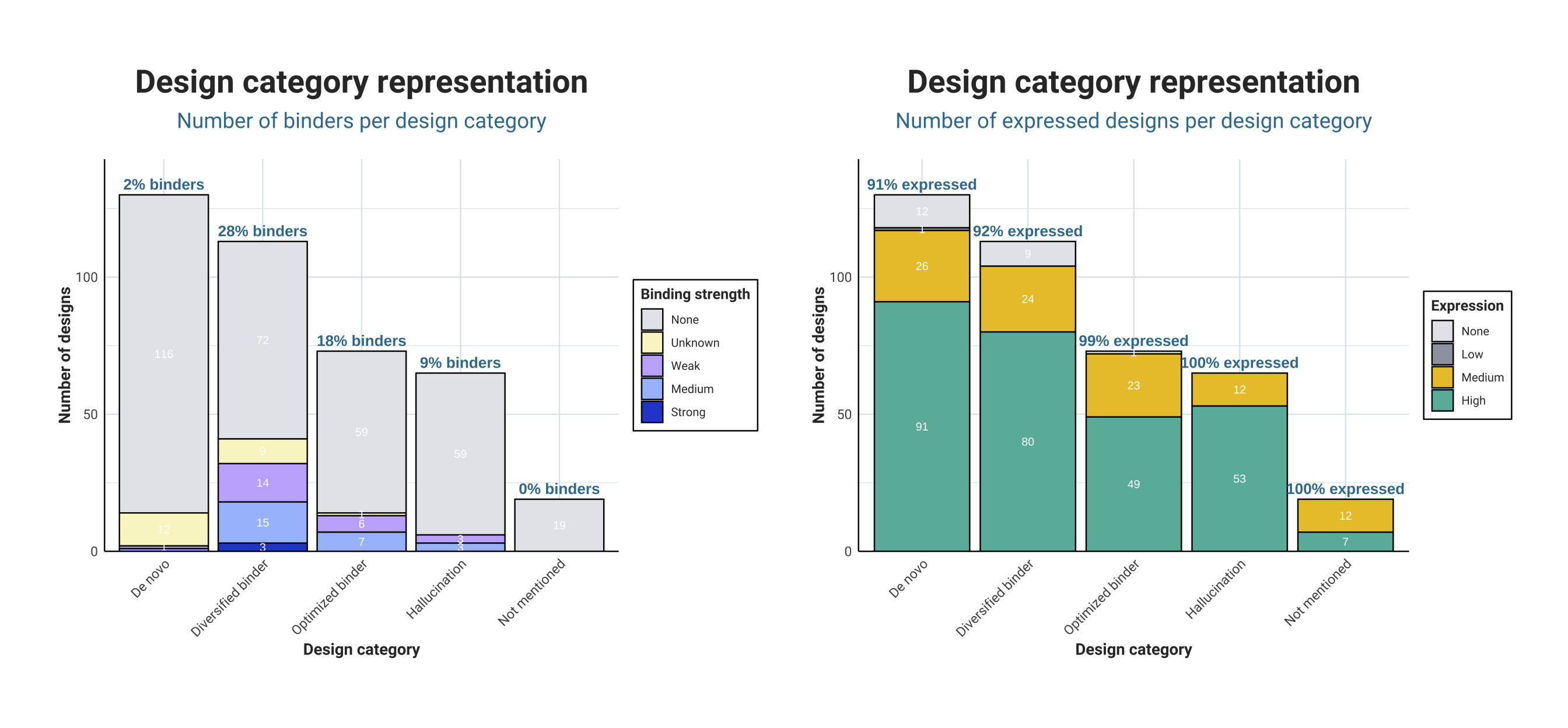
We’ve talked before about how popular the diversified and optimized binder choices were for landing the top 100 spots. Most of these were submitted within a couple of days from the deadline. And yet, all designers choosing these were rightfully doing so: we have a 28% hit rate for diversified binders and 18% for optimized. We’ve already seen how successful taking an existing binder and redesigning its non-interface regions was: Cradle got a better binder than Cetuximab, Chris Xu had a few strongly binding ones, and Aurelia Bustos had an 80% hit-rate. The message is clear: if you want to create a better binder, it helps to have an existing one to start from!
The expression rate for those diversified binders is quite surprising: 92% and thus lower than hallucination and on par with other de novo methods. We think some people departed too far from the natural sequence for this, trying to maximize the scores. Yet, this is something worthy of a deeper investigation.
For de novo, hallucination (BindCraft) got 6 out of the 7 binders, with diffusion (RFdiffusion) claiming the final one. The overall success rate of hallucination (9%, 6/65 tested, as seen in the bar plot above) is on par, if not slightly lower, than reported for other targets in the BindCraft paper. All of them expressed, showcasing what a difference the SolubleMPNN redesign step makes. Along with filtering for expression using tools like NetSolP, these pipelines and the reliable high expression rate across all algorithm categories make us say claim that expression has basically been solved.

We also wanted to see whether the new metrics had an impact on the number of resulting binders, or if a random selection is still better. Ultimately, we see a stark difference: 24% of the top 100 designs were binders, whereas only 10% were from our selection maximizing model diversity. The causal relationship is still blurry. Did any of these metrics (ipTM, iPAE, ESM2 PLL) play a actual role in predicting binders, or were the top 100 approaches that mostly diversified natural binders like TGF$\alpha$ and EGF bound to bind already, and landing in the top 100 was just a side-effect?
We could assume the metrics helped with expression, given the higher rate in the top 100. But the question remains the same: is there a correlation between metric optimization and expression rates, or between the metrics and binding? We’ll investigate these 2 questions next.
Did our selection have any influence on the binding affinity?
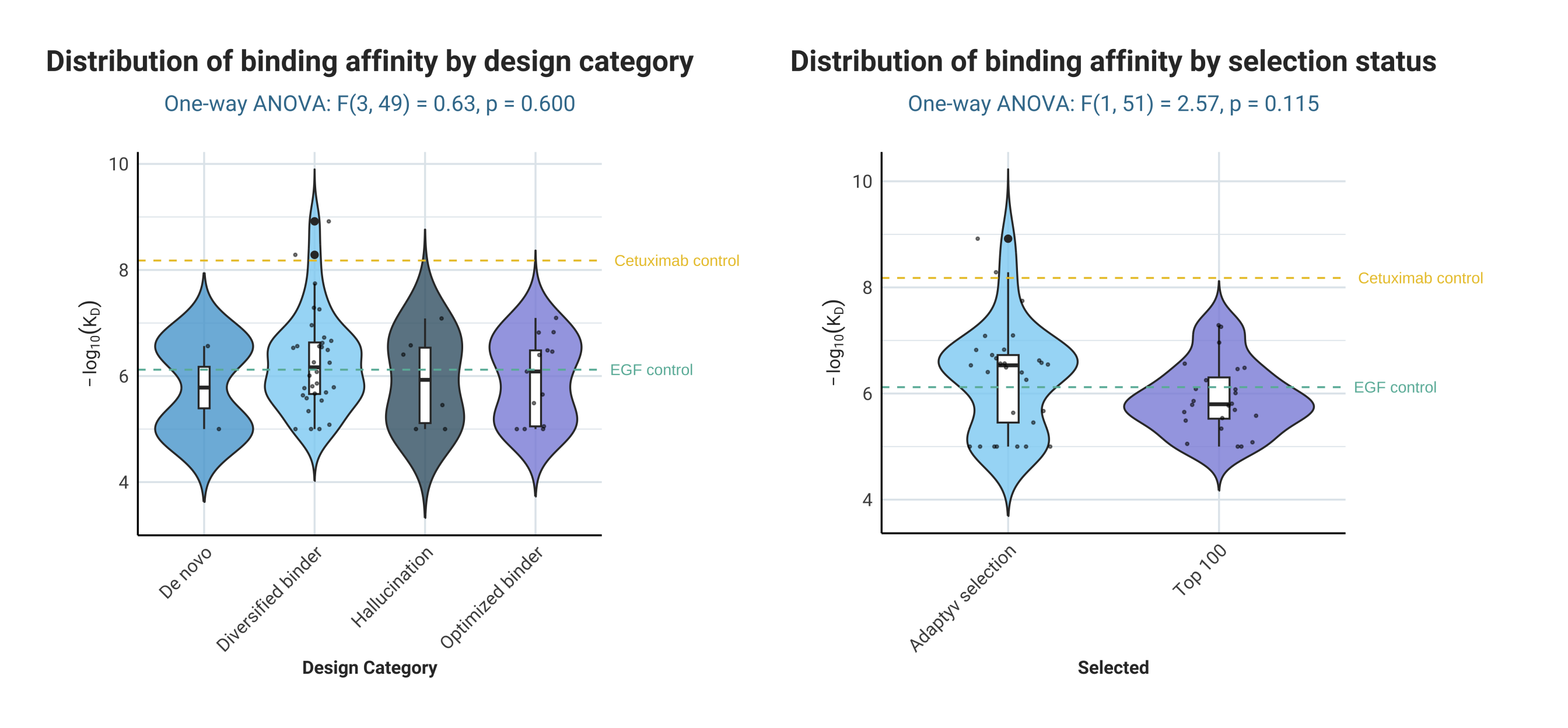
There is almost no difference when looking at the distribution of binding affinities per design categories. The median values are similar and, when performing an ANOVA test on the mean values, there is no significant difference. At most, we see some outliers - Cradle and Chris’s binders outcompeting Cetuximab’s binding affinity.
Some differences arise when comparing the $K_D$ per selection status (distribution shifts, slight differences in medians), yet there is still not statistical significant when comparing the mean values with an ANOVA test. It is interesting that the pseudo-random selection has a higher affinity range. There are some aspects we’ve skipped in this analysis, mainly the normality assumption. We can see how the Adaptyv selection category forms an almost bimodal distribution, with the designs in the 1e-6 M likely originating from EGF/TGF$\alpha$, and in subsequent analyses we can account for both the design origin (or de novo) and selection status. But, we can highlight our main conclusion: landing in the top 100 w.r.t in silico metrics played no detectable role in increasing the binding affinity.
Evaluating the in silico scores: we’re still at the beginning
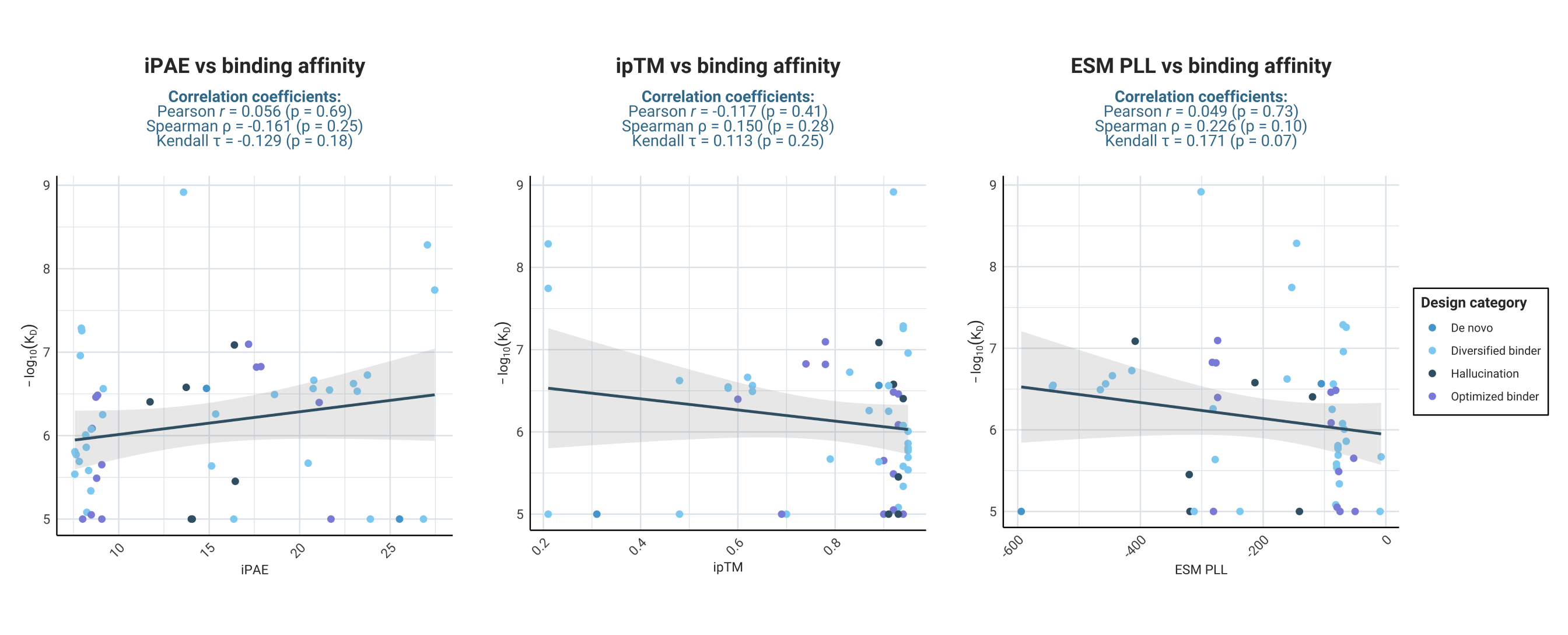
Let’s look more directly into the correlation (or lack thereof) between our chosen scores and binding affinity. In all cases, we find no significant correlation for any of the metrics. For most, the trend is quite peculiar: we would expect and increase in $K_D$ as iPAE decreases, as ipTM increases, or as ESM PLL increases, yet all trends are the opposite. We also need more data points for a conclusive statement, as we are likely undersampling our binders.
Thus, we cannot ascertain if any of these scores are meaningful when modulating the continuous affinities. But, are they predictive of binding or expression as binary labels?
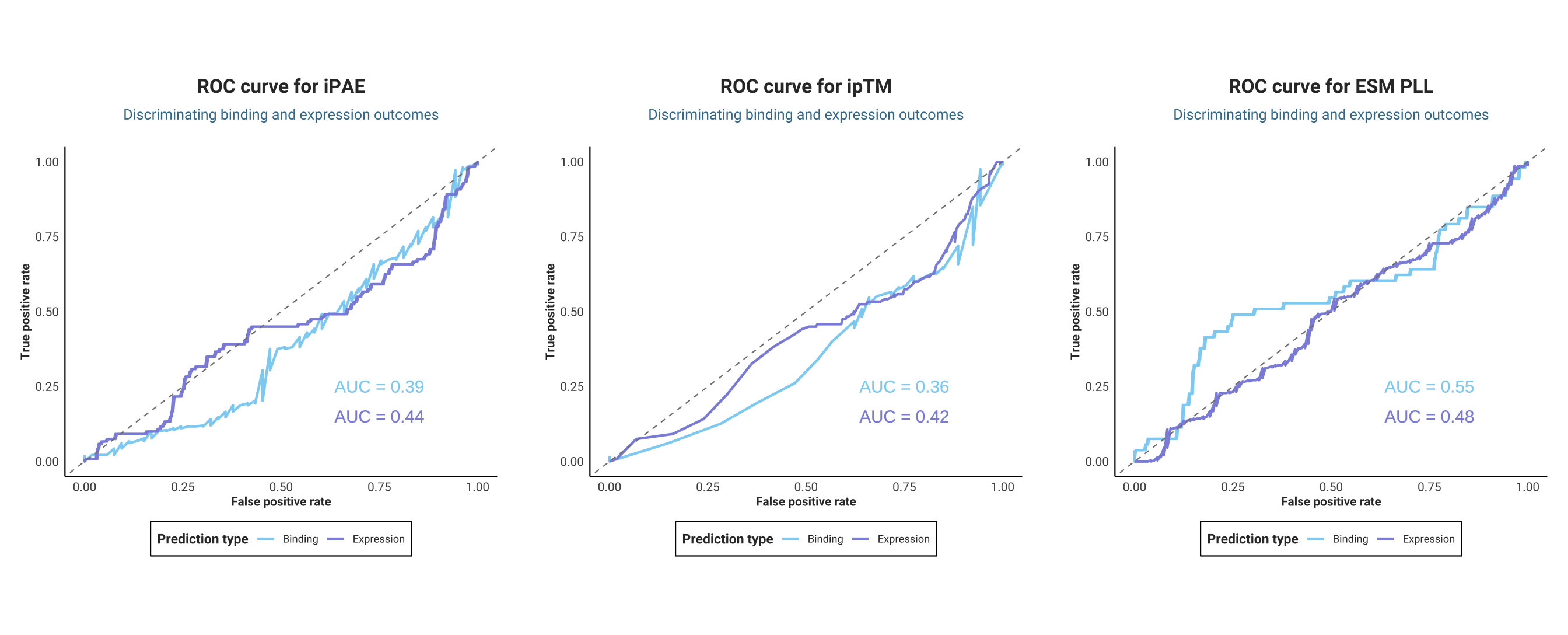
From the figure above, the definite answer is “No!”. When plotting the ROC (receiver operating characteristic) curves and calculating the area-under-the-curve (AUC), most values range in the 0.5 region (random classifier, meaning these metrics cannot discern between binding and non-binding or expressing and non-expressing designs better than a coin-flip). Some metrics are better predictors. Leo Castorina and other people from the Wells Wood lab showed that scores like %identity to PDB and TMscore are better predictors, with an AUC above 0.6, while glutamic acid or lysine composition can discriminate highly expressing from weak or non-expressing designs. They computed even more scores that could correlate with expression or binding using their DE-STRESS tool, and you can find all of them here. We thank the people from the Wells Wood lab for their active involvement in this competition and manuscript preparation!
It’s interesting that the normalized ESM pseudolikelihood (divided by the sequence length) is one of the better binding predictors, with an AUC of 0.72. As mentioned in our consortium discussions, it is likely due to the several binders being modified versions of existing ones, towards which ESM2 would be biased.
All tested designs formed 3 main classes: EGF or TGF$\alpha$-like, antibody-like, and de novo/others

Previously, we noticed a homology-based clustering for the designs starting from EGF and TGF$\alpha$, yet other clusters were not as distinguishable. For only the tested designs, new clusters for antibody-like and de novo-like designs appear. The antibody-like cluster contains all strong binders. We cannot discern a separation by binding strength or expression level in the ESM2 embedding space, although it seems the antibody-like cluster had more non-expressing designs. For a more in-depth analysis, looking at the percentages of binders or expressed designs per starting point is key!

Similarly, in the structure-informed SaProt space, we find the same clusters. This time, there is a higher overlap between EGF and TGF-like designs, and a more continuous distribution for the other designs or de novo. We still cannot discern specific expression or binding clusters, although it seems the EGF-like region is more biased towards medium expression designs. Thus, it’s still an art to adequately shape-up a known binder, optimize it, and ensure it will still express or bind.
Domains I and III of EGFR were the preferred targets
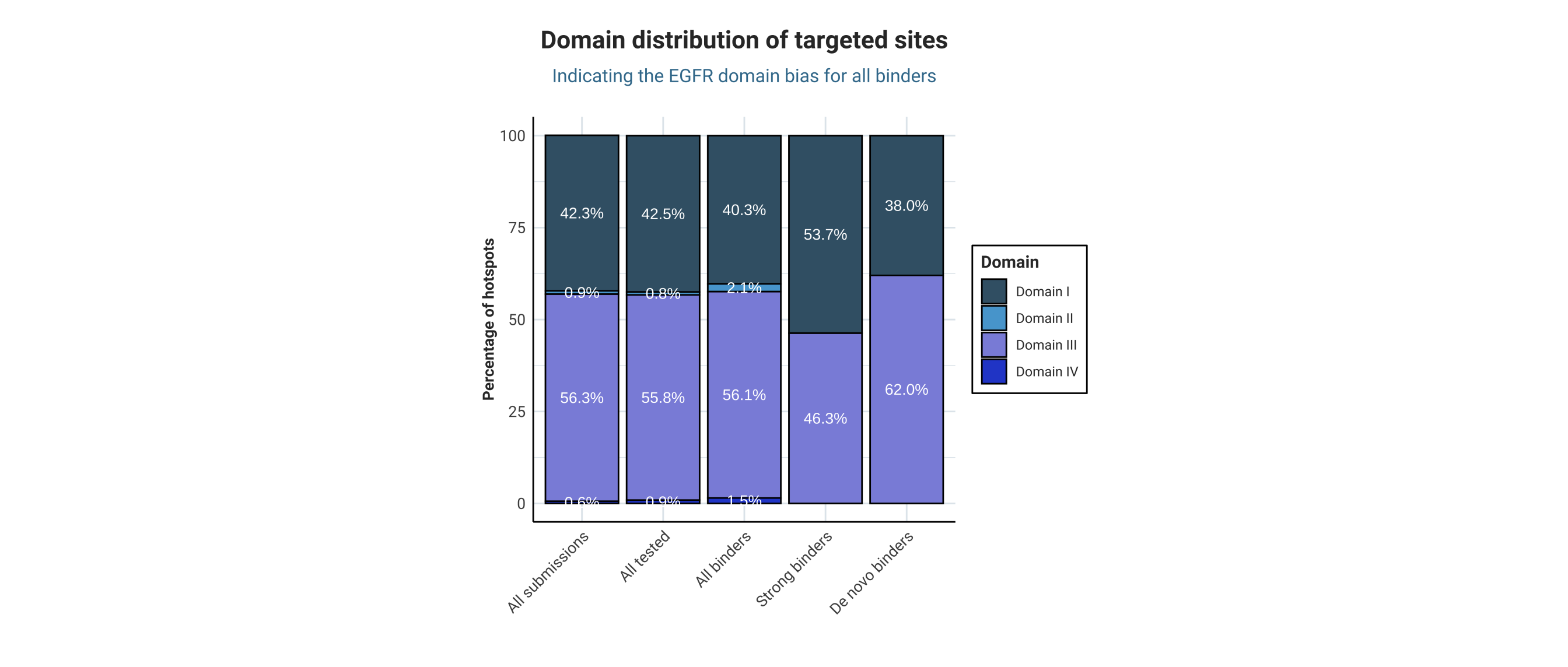
We advised you to select a specific EGFR epitope (residues 11-13, 15-18, 356, 440-441), at the interaction between Domains I and III of EGFR. We believe this is reflected in the targeted site distribution per domain, especially for all submissions and all tested ones. Domain III is consistently the most targeted region, accounting for about 46-62% of binding sites across all categories. Interestingly, this preference is most pronounced in de novo binders (62%), but less so for the top 3 strong binders (46%), even though Cetuximab interacts strongly with Domain III, while EGF binds to the aforementioned hot spots. Moreso, some binders preferred Domains II and IV as well, albeit at insignificant percentages.
This poses even more questions we are unable to address in our exploratory analysis. Why do de novo binders prefer Domain III? Which sites are exactly targeted by starting point or de novo? Is there a large deviation between original and modified binder sites in the diversified or optimized cases? We invite you to join our consortium if you have answers for these questions.
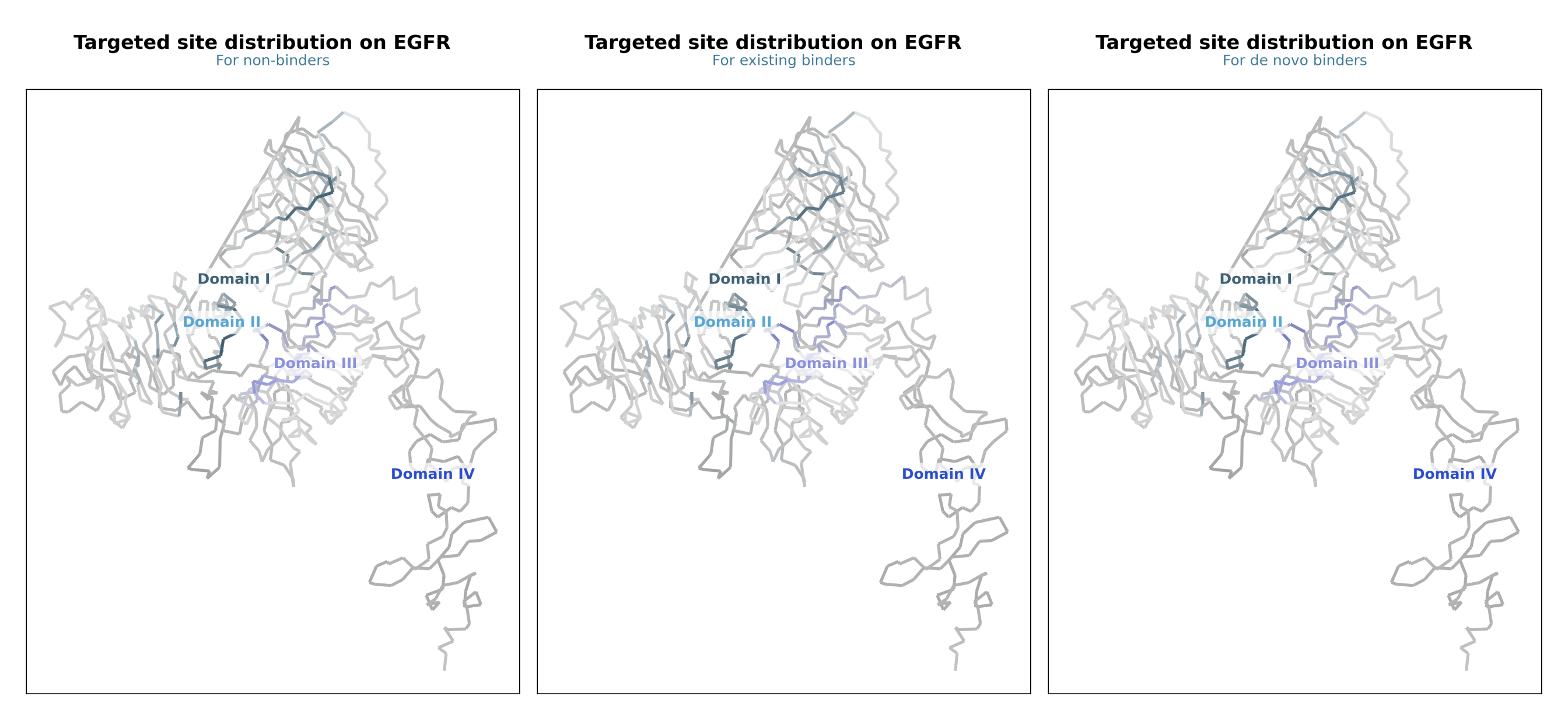
We illustrate the same patterns with these pseudo 3D EGFR plots (thanks to ColabDesign for its implementation), this time separating binders as de novo or from an existing starting point via a homology search using MMseqs2. We see the same trend: Domains I and III are the preferred targets.
Glutamic acid is more prevalent in de novo designs’ interfaces
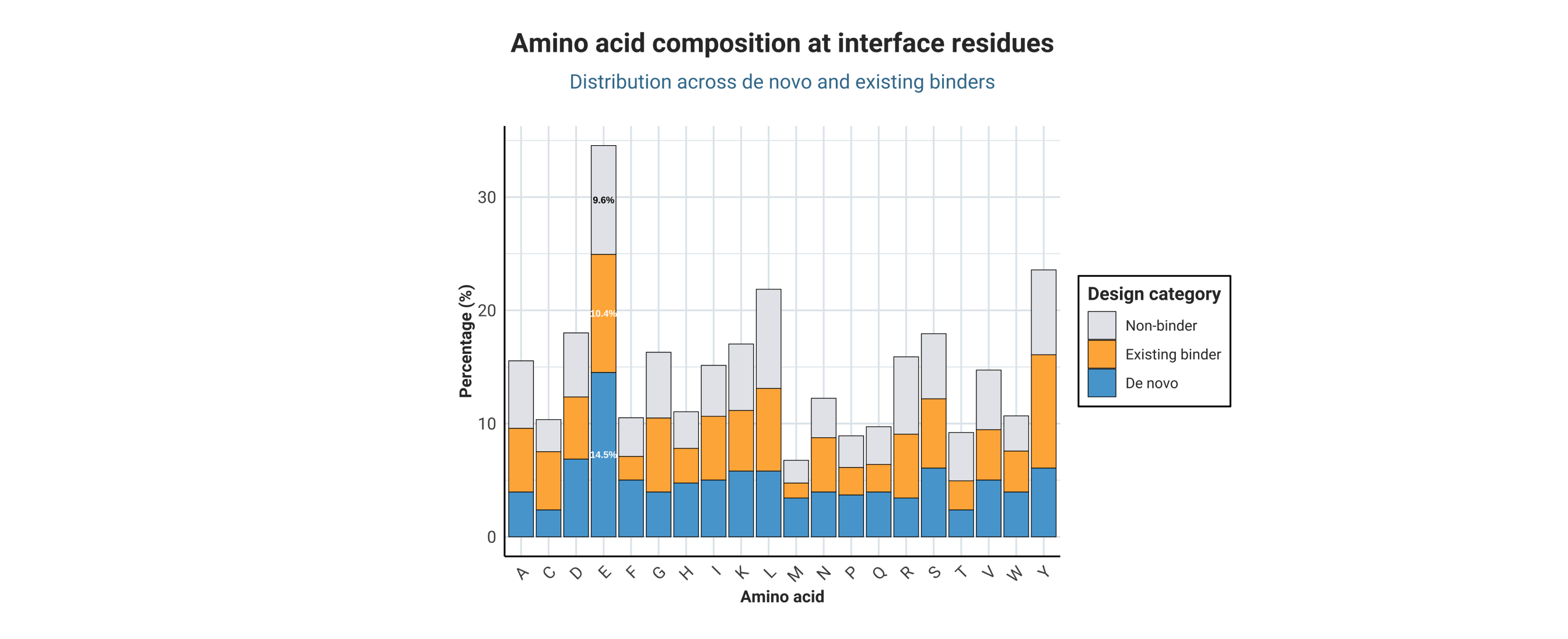
We next wanted to see if the amino acid composition of binding sites differs between non-binders, de novo binders, and designs starting from known ones. The main difference is for glutamic acid (E), a hydrophilic amino acids, which has a higher frequency in de novo binders (14%) compared to the other 2 classes (around 10%). We assume this is likely due to the SolubleMPNN redesigning step most de novo methods used to improve solubility and expression, which biases proteins towards more hydrophilic surfaces (thus binding sites as well). Glutamic acid composition is also an effective predictor of expression, as we mentioned above.
There are other amino acid preferences for de novo binders, such as methionine (M) and phenylalanine (F), both hydrophobic amino acids, yet these occur at lower frequencies.
The interface properties are more indicative of the true binders
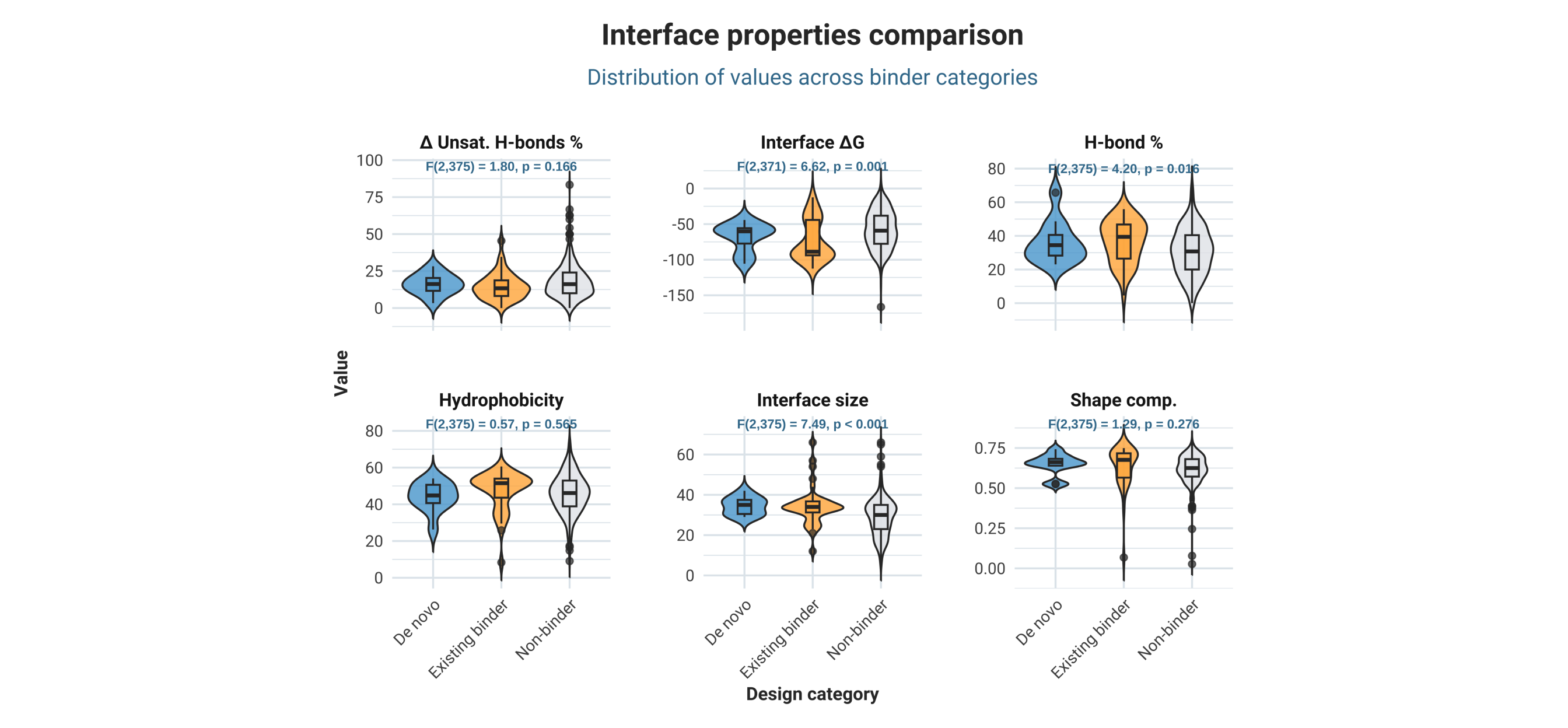
We next investigated the interface physicochemical properties of binders versus non-binders. The main differences between binders and non-binders are for the interface $\Delta{G}$, the percentage of hydrogen bonds, and the interface size, all statistically significant following an ANOVA test. Unsurprisingly, keeping an existing binding site yields the lowest interface $\Delta{G}$, whereas de novo is in close range to the non-binder ones. De novo proteins, in turn, have more interface contacts compared to non-binders. We have not found any significant differences for the interface hydrophobicity, nor the shape complementarity between binding site and epitope.
We can now see why both the diversified and optimized binder approaches were so popular: keeping an existing binding region with adequate predicted binding free energy, then simply scaffolding it in a backbone that optimizes the set of metrics we’ve chosen, seemed like the most straight-forward approach to both generate binders and land in the top 100.
The top 5 de novo binders and existing ones have some interface property overlap
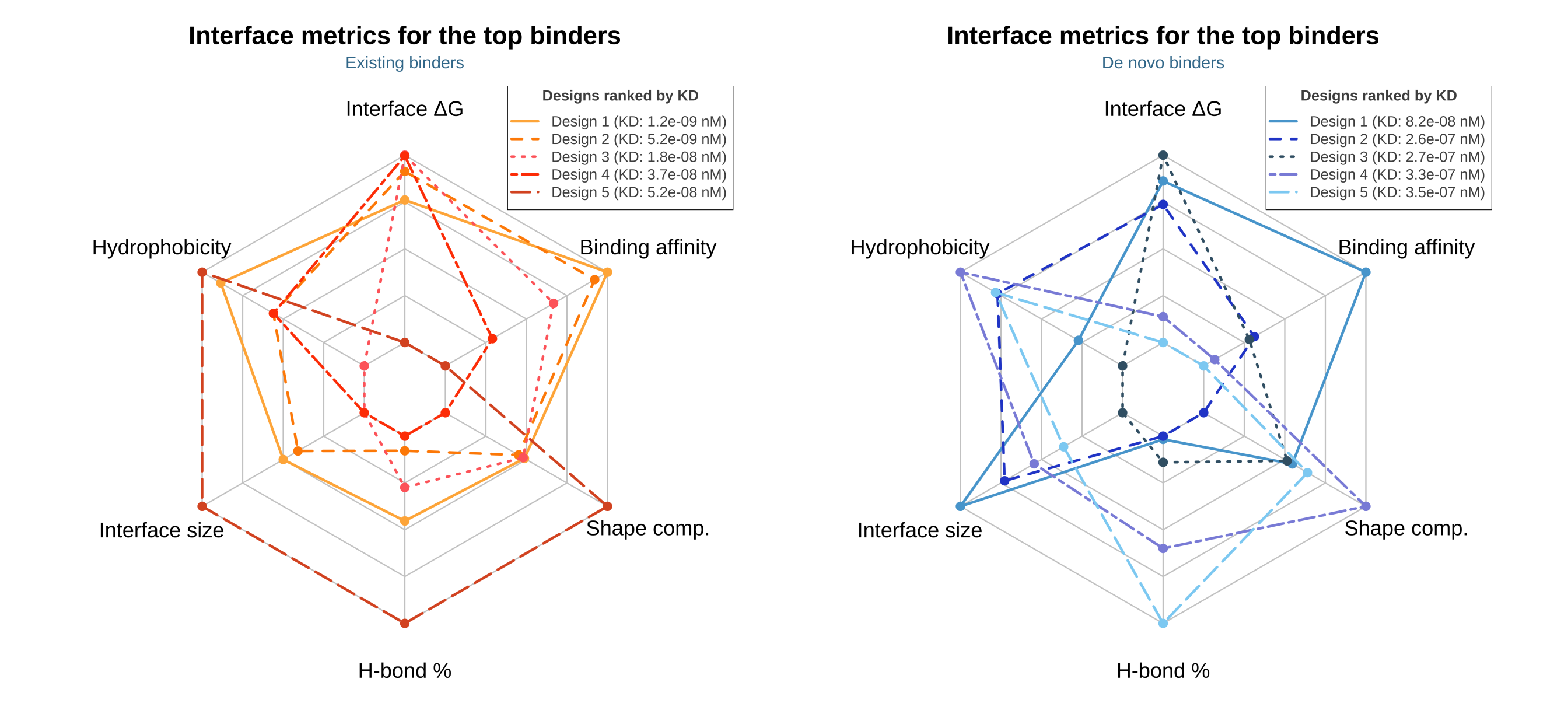
Finally, we investigated if the top 5 de novo designs (as ranked by $K_D$) differ significantly from the top 5 designs starting from existing binders. The most striking difference appears in their interface sizes - most of the top-ranking de novo designs aim to increase the number of interface contacts. We assume this is due to BindCraft’s (used in 6/7 de novo binders) loss function that accounts for this. Both de novo and existing starting point methods achieve high interface $\Delta{G}$ values. De novo designs show consistency in their interface properties, especially size and energetics. Meanwhile, the existing binder designs display more variability, likely due to their diverse evolutionary origins.
Interestingly, no single design optimizes all interface properties simultaneously. Some excel at shape complementarity but show average hydrogen bonding, while others achieve great energetics with moderate hydrophobicity scores. This is evidence of the fact that (except for filtering based on predicted $\Delta{G}$ or maximizing the number of contacts), there is no secret recipe for binder designs and several of these methods optimize different properties.
Conclusion: Has protein binder design been solved?
Not yet — but the rate of progress in protein engineering is astounding. Within just a few months from Round 1 (September) to Round 2 (December), we witnessed dramatic improvements in expression and binding success rates, along with a surge in participation from the protein design community.
Generating soluble proteins – those that express and fold well – seems to be essentially solved, at least in our small-scale bacterial cell-free expression systems. The next challenge here will be to figure out how to translate expression between different organisms to ensure high production yields in larger reactions so that all those novel proteins can be put to good use outside of lab-scale testing. Luckily, people are already thinking about generating datasets for predictive models of expression like e.g. at Align to Innovate.
Optimization of existing binders is proving remarkably effective and can systematically yield better proteins than previous candidates. Cradle's success in this competition demonstrates how this approach is now being rapidly industrialized and we hope many applications can benefit from such tools.
In de novo design, BindCraft has brought about a significant increase in success rates and has immediately been adopted by the protein design community. It remains to be seen whether this represents a broader shift toward hallucination models outperforming diffusion approaches, or if the next generation of diffusion models will shift the meta back.
Both in this competition and across the field, we still struggle to predict binding success through computational metrics. This gap between the ability to generate candidates and predict their performance represents one of the field's most pressing challenges and we need to generate more data to build better models here.
Thanks again to all participants for making this competition an immense success through sharing your cool designs and interesting approaches. We have exciting plans for 2025 and can't wait to see what kinds of proteins you'll design next.
If you want to participate in a paper write-up in the coming weeks about the two rounds of the Protein Design Competition, you can fill out this form to join.
If you want to stay in the loop about future competitions and releases from us, leave your email here!
About Adaptyv
Adaptyv is the cloud lab for protein designers. Our platform is the fastest way for you to experimentally validate your protein designs. Just select an assay, upload your sequences and get high-quality experimental results in under 3 weeks. Check out our pricing here or just email us at hi@adaptyvbio.com to set up your data generation campaign!